WDC - Weighted Degree Centrality
Definition
In WDC, the gene expression profiles are incorporated into the PPI network in terms of Pearson
correlation coefficient and develope a essential protein prediction method based on PCC and ECC.
Computational prediction of essential proteins based on the global protein-protein interaction (PPI) network is severely restricted because of the insufficiency of the PPI data, but fortunately the gene expression profiles help to make up the deficiency. In WDC, Pearson correlation coefficient (PCC) is used to bridge the gap between PPI and gene expression data.
The weighted degree centrality WDC of a node i is the sum of weighted values of the edges connecting node i and its neighbors. It can be expressed as:
where Ni is the set of neighbors of node i and Wi,j refers
to the weighted value of edge between node i and its neighbor j.
The weight of an interaction is given by the metric described as:
The performance of WDC is better when λ∈ [0.3, 0.5]. Its performance in particular is optimal when λ = 0.5.
Computational prediction of essential proteins based on the global protein-protein interaction (PPI) network is severely restricted because of the insufficiency of the PPI data, but fortunately the gene expression profiles help to make up the deficiency. In WDC, Pearson correlation coefficient (PCC) is used to bridge the gap between PPI and gene expression data.
The weighted degree centrality WDC of a node i is the sum of weighted values of the edges connecting node i and its neighbors. It can be expressed as:
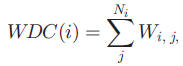
The weight of an interaction is given by the metric described as:
W = ECC × λ + PCC × (1-λ)
The performance of WDC is better when λ∈ [0.3, 0.5]. Its performance in particular is optimal when λ = 0.5.
Software
References
- TANG, X., WANG, J., ZHONG, J. & PAN, Y. 2013. Predicting essential proteins basedon weighted degree centrality. IEEE/ACM Transactions on Computational Biology and Bioinformatics, 11, 407-418.